- 16 juillet 2024
- Mistral AI team
We’re contributing Mathstral to the science community to bolster efforts in advanced mathematical problems requiring complex, multi-step logical reasoning. The Mathstral release is part of our broader effort to support academic projects—it was produced in the context of our collaboration with Project Numina.
Akin to Isaac Newton in his time, Mathstral stands on the shoulders of Mistral 7B and specializes in STEM subjects. It achieves state-of-the-art reasoning capacities in its size category across various industry-standard benchmarks. In particular, it achieves 56.6% on MATH and 63.47% on MMLU, with the following MMLU performance difference by subject between Mathstral 7B and Mistral 7B.
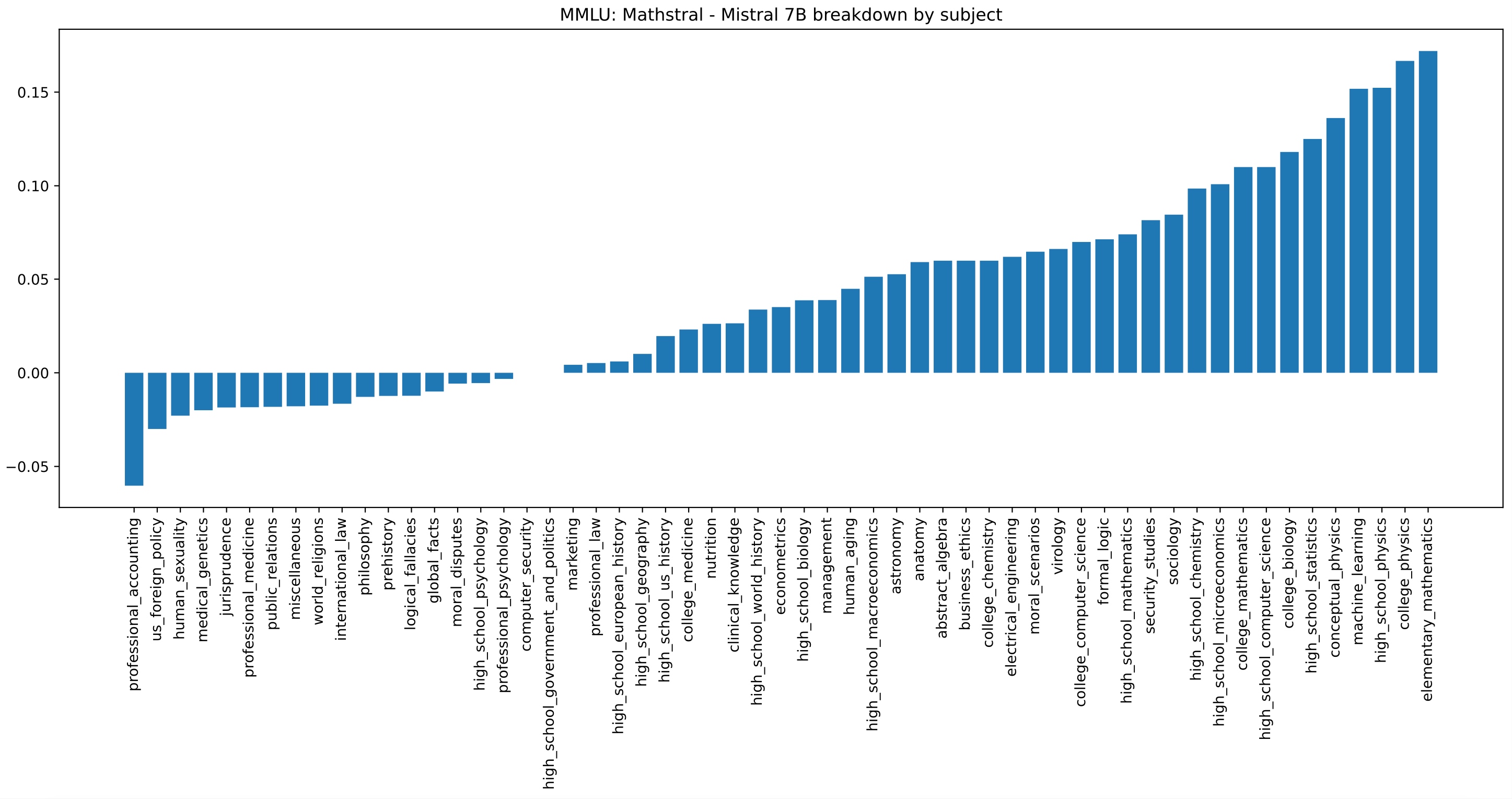
Mathstral is another example of the excellent performance/speed tradeoffs achieved when building models for specific purposes – a development philosophy we actively promote in la Plateforme, particularly with its new fine-tuning capabilities.
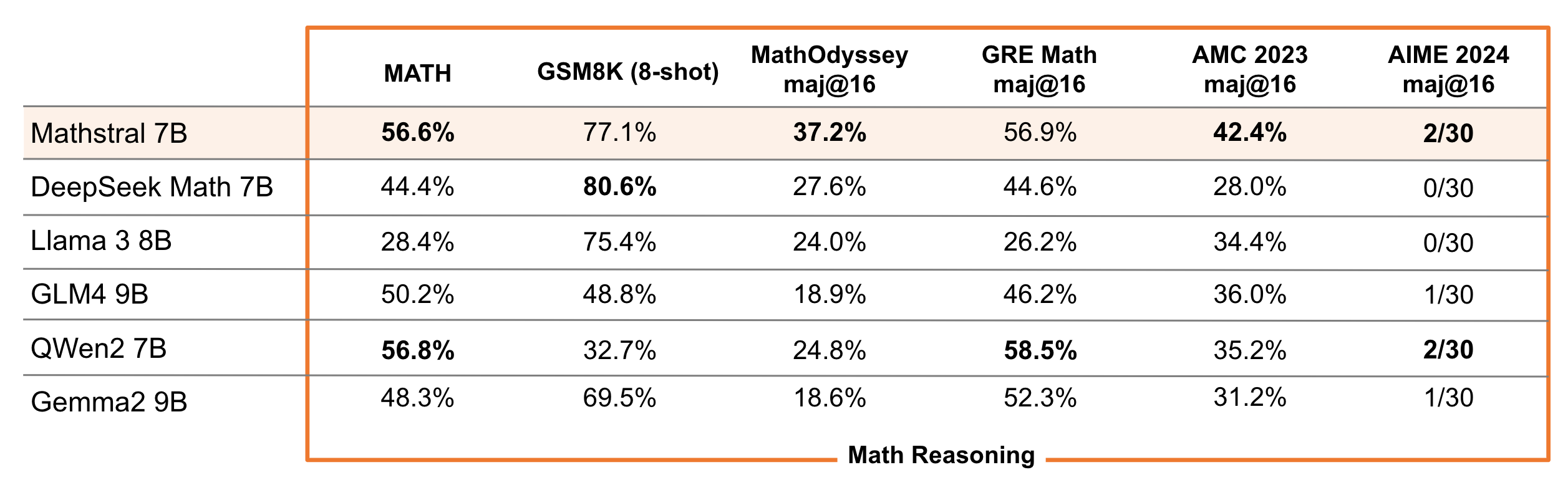
Mathstral can achieve significantly better results with more inference-time computation: Mathstral 7B scores 68.37% on MATH with majority voting and 74.59% with a strong reward model among 64 candidates.
Mathstral is an instructed model – use it or fine-tune it as such, referring to our documentation. Weights are hosted on HuggingFace. You can try Mathstral now with mistral-inference and adapt it with mistral-finetune.
We thank Professor Paul Bourdon for curating the GRE Math Subject Test problems used in our evaluation.